Why you can’t ignore master data management and data governance

This is the second in a series of four posts on breaking down data silos and its importance for organizations. In this post, we look at master data management and data governance strategy. In our first post in this series, we discussed why it’s not ideal for your overall data program to be primarily driven by out-of-the-box business intelligence (BI) and visualization tools. After all, the credibility of your data program rests on having actionable, repeatable and accurate data across the organization. Adhering to data governance best practices is essential. And while popular BI software is excellent for working with cleaned, integrated data for ad-hoc and one-off reporting, it’s not always the best solution for implementing a master data management (MDM) strategy where a single version of the truth is required across an organization. So what does a successful MDM strategy look like? Master data management explained MDM is the process of defining and managing all your organization’s critical data, no matter which department or sector it’s from, and linking it all to one master file. When combined with data integration, good MDM strategy ensures the entire organization agrees on basic principles such as definitions and rules, while ensuring everyone works from the same master dataset for repeatable, accurate analysis and insights. MDM establishes cross-organizational consistency and control of data across departments, so the entire entity uses the same processes for collecting, aggregating, quality assuring and distributing or displaying data. MDM tools establish processes for data cleanliness by removing duplicates, unifying data types and cutting out irrelevant or inaccurate data (which can grow exponentially if your organization’s culture encourages practices like data siloing ). When well executed, MDM streamlines and encourages data sharing among departments within an organization. It also ensures the organization doesn’t use multiple versions of data in different departments. MDM and data governance According to Dataversity, data governance is the term for the set of disciplines that underpin the success of an MDM program. These components include things like data architecture management, data quality management and data warehousing/ business intelligence management. Unfortunately, MDM and data governance strategies are often poorly executed or ignored by organizations, either because the organization hasn’t made the necessary cultural shift, or they don’t have the technology needed to execute on a culture of data sharing. In the retail space, this can take the form of marketing emails sent to a client about a product after that client has already purchased the product. SaaS companies with bad MDM strategy routinely offer employees from competing companies software demos (and, therefore, inside information), simply because these employees were at some point automatically added to a siloed marketing list. MDM and M&A One of the most common instances of chaotic data management lies in mergers and acquisitions , when one company acquires the data (and other assets) of another firm. While database admins can usually marry much of the data between companies, the particular complexities of each system mean they’re typically never fully integrated. This can lead to instances of database admins being forced to maintain multiple databases and, therefore, multiple versions of the truth. As more M&A deals are done and more data is acquired, this problem can quickly escalate for an organization if not addressed head-on. Culture shift At its core, implementing an MDM strategy is about much more than data or technology. It’s also about changing your organization’s culture. With this in mind, Information Management names ten data governance best practices when creating and executing your strategy:
- Establish a business case. Link your MDM strategy’s success to the organization’s overall business goals.
- Get executive buy-in. Having a champion in the C-suite in your back pocket can do wonders if things get political or conflicts arise.
- Get business groups involved. MDM strategy often dies on the vine if users aren’t excited or, worse, uninformed. Get business users involved early and keep them engaged.
- Fail to plan, plan to fail. Don’t start an MDM strategy half-cocked: devote sufficient planning before implementation, or risk failure.
- Nominate a governance committee. Do this in advance of implementation: strong leadership can go a long way in advancing a centralized data program.
- Choose the right architecture and topology. Every organization’s MDM strategy is unique, and so are their topology and architecture needs.
- Define your data quality strategy. This is crucial since one of the main goals of MDM is to improve data quality.
- Retain the right staff and SI partner. The ideal team is a mix of technical and business experts from both within your organization and outside consultants.
- Go gradually. Implement an iterative approach to your centralized data program, or risk turning off users by going too fast, too soon.
- Tackle low-hanging fruit first. A quick win out of the gate can win hearts and minds across the organization, while also boosting morale.
Share this
Previous story
← HugePages for Oracle Database in Azure Cloud
You May Also Like
These Related Stories
Achieving data integration zen: building your business case

Achieving data integration zen: building your business case
Jan 20, 2019
3
min read
Breaking down data silos: BI’s broken data integration promise
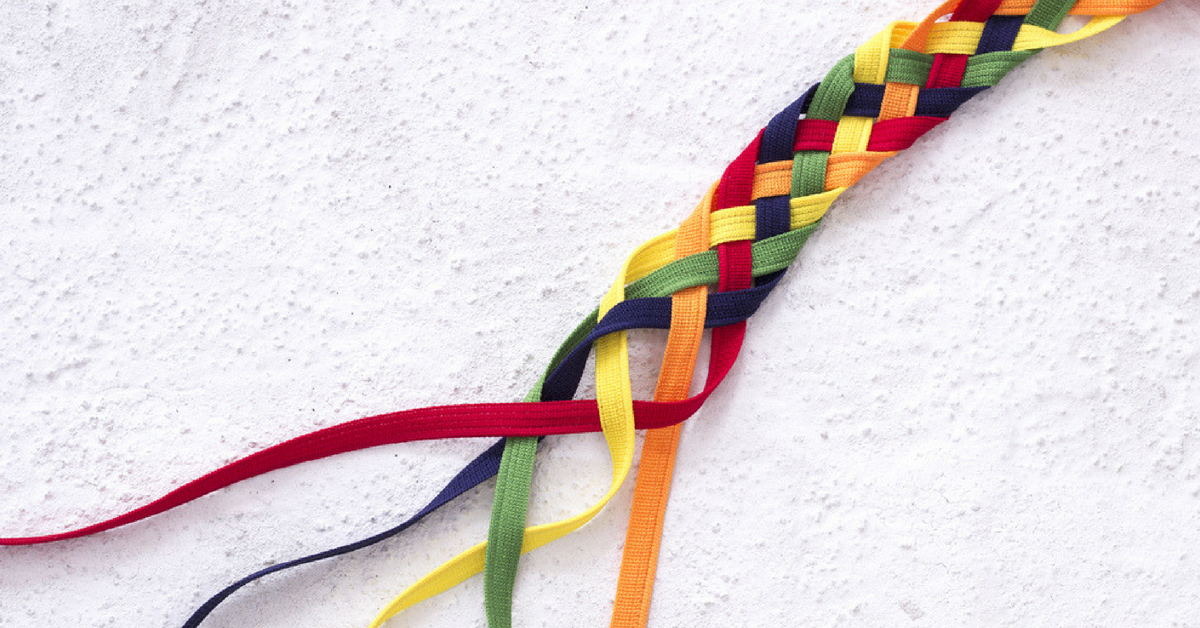
Breaking down data silos: BI’s broken data integration promise
Aug 16, 2018
4
min read
Dismantling data silos through cloud integration

Dismantling data silos through cloud integration
Feb 23, 2019
3
min read
No Comments Yet
Let us know what you think