Tips that Help Alleviate Pressures on DataOps
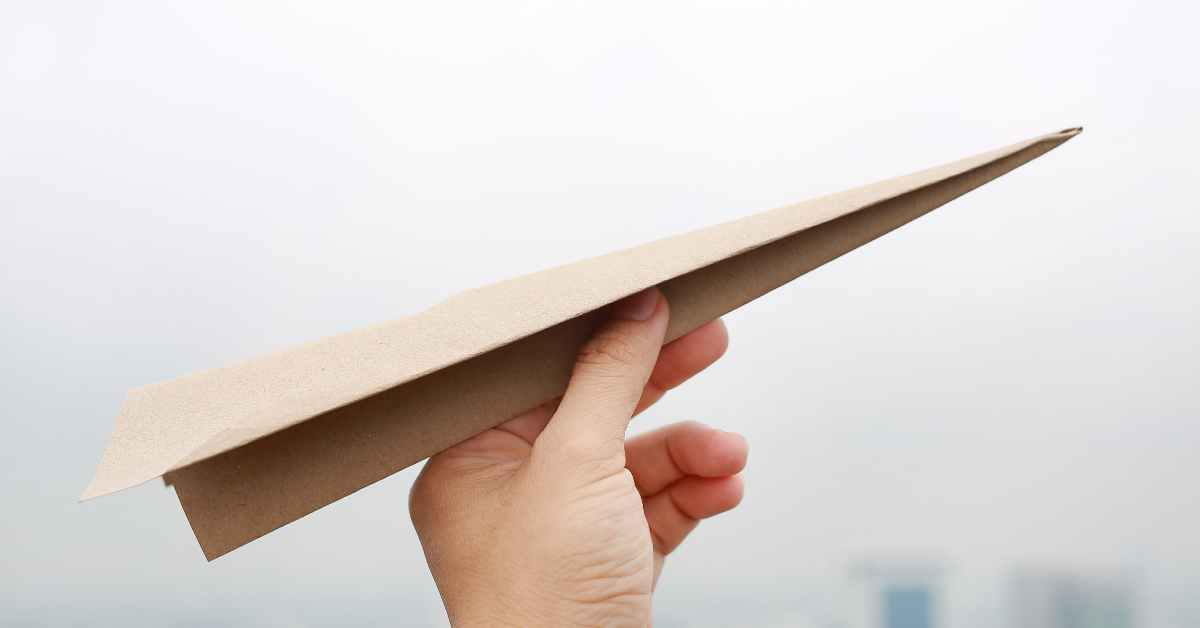
Data is hard. Its always been hard, and it’s not getting easier. We always have more of it, there are more sources to integrate, it changes all the time, the quality is questionable, and the business wants it all right away. Working at this pace requires a sound operational mindset to avoid driving your teams crazy once the business starts using the data. This mindset needs to develop very early in every data project to ensure that you can keep your operational costs at a minimum and, most importantly, enable teams to easily maintain the data moving forward. So how do you alleviate the pressures on DataOps teams? It comes down to four key components:
- Resiliency
- Instrumentation
- Proper alerting hygiene
- Client visibility
Resiliency
One of the most important things you can do is ensure that what you create is built with resiliency in mind. I'm not talking about infrastructure redundancy or auto-scaling but rather the end-data product that the business is using. In other word, the data should always be in a usable state. You might not always have the latest, but what you do have is complete and accurate. One typical example is a daily traditional batch full refresh of a data source. I don’t know how many times I’ve seen this scenario:Job Steps | Business Impact |
Truncate the target data set. | No data available until the load is finished. Completely unusable. |
Bulk load the data (can take minutes to hours). | No data available until the load is finished. Completely unusable. |
Outcome: Success. | Usable data again. |
Outcome: Error. | Empty or inconsistent data set. |
Instrumentation
The more metadata you have about the pipeline execution and quality of the data being loaded, the better. It allows you to incorporate proper metrics and business KPI's into the pipeline code, speeds up troubleshooting, enhances trending analysis, and (once you're good) predict failures with ML modelling. This should provide a better experience for the business and allow your DataOps team to focus on data changes or new data sources as opposed to spending an inordinate amount of time fixing existing issues.Proper Alerting Hygiene
You don’t want to be "alert happy." If you are, you won't retain your DataOps team for very long. You want to get their attention only when it matters. As such, you should not alert them to every error or even necessarily look for errors. You want to get their attention when it affects the business. For example, alerting on the failure of a job performing an incremental update every five minutes doesn't matter if all you really care about is the successful execution of the same job once an hour (if that's acceptable by the business users). Those errors should be investigated from a trending point of view, but you should not look at all of them as they happen. When you do trigger an alert, make sure that the alert is clear, concise, easy to understand, and actionable. There's nothing worse than getting a massive trace dump then expecting a DataOps team member to dissect it and resolve the issue while business users continually ask, “is it fixed yet?” When it comes to alerts, it's essential that you know about a problem before the business users do. There's nothing worse than having a senior business executive call you to tell you that their data isn't available just to discover out that you were blissfully unaware. Always set up your alerting with the business in mind.Client Visibility
This one is relatively easy but goes a long way into building trust with your business users. Make sure that people who use the data to make decisions and create reports know the state of the data at all times. Metadata around the recency and quality of the data should be made available and incorporated into the semantic layers used by the various reporting, business intelligence, and data visualization tools. The additional benefit is a reduction in inquiries to DataOps asking whether the data is up to date. If people trust the data, they will use it. Otherwise, they won’t. It’s that simple, and it happens quickly. We are just scratching the surface here. We can talk about scenarios and techniques for days. Hopefully, this post helps you get a head start if you're beginning a data pipeline project.Share this
You May Also Like
These Related Stories
8 Questions To Ask When Choosing A Database Managed Service Provider
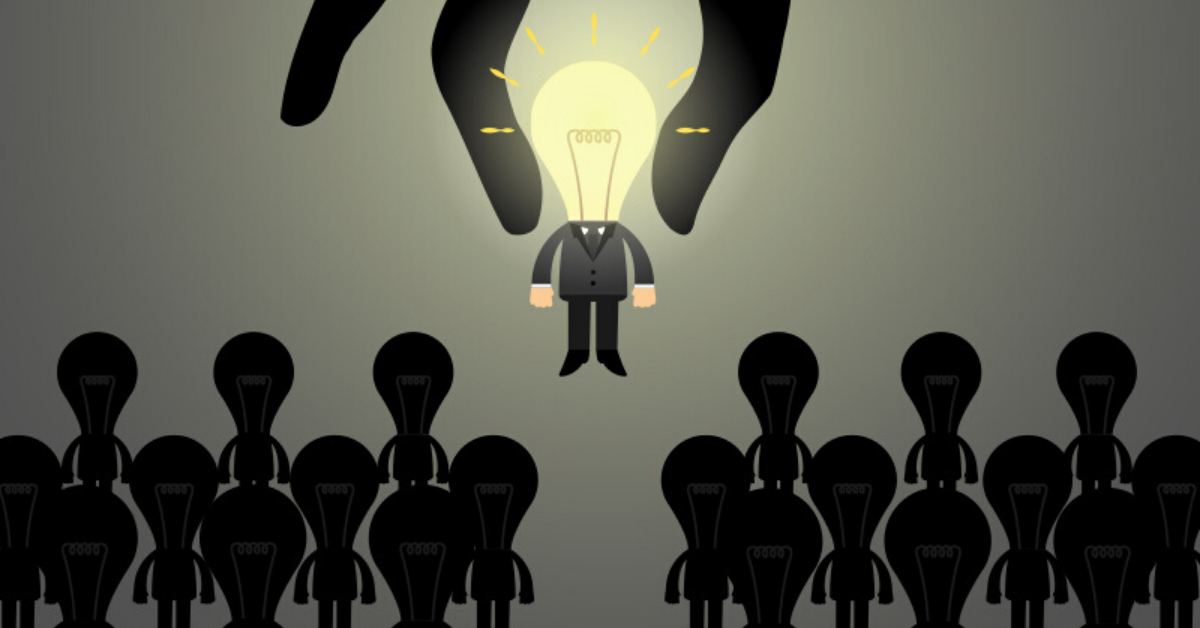
8 Questions To Ask When Choosing A Database Managed Service Provider
Jul 6, 2020
3
min read
First touch penalty on AWS cloud
First touch penalty on AWS cloud
Mar 26, 2018
6
min read
Is the DBA career dead?
Is the DBA career dead?
Jan 28, 2016
4
min read
No Comments Yet
Let us know what you think