The Developing Data Literacy Crisis

Failed data-focused initiatives are not new. Since the beginning of our journey to empower organizations to leverage data we have seen data warehouse projects go off the rails, data lake initiatives fail to achieve their ROI, and visualization initiatives come crashing down with low user adoption or lack of trust in the results. This is a common part of technology evolution, we must learn the proper techniques and practices to apply to different types of organizations, ensuring that we address past traumas and proactively address organizational concerns. The failed initiatives I have seen have often been a result of one or more of these four primary reasons:
- Requirements Clarity - Many early data warehouse projects struggled due to a lack of clarity on requirements. Requirements were either so specific as to never be built or so broad as to prevent alignment across stakeholders on what success meant.
- Clear Prioritization - Data lakes began with a vision to integrate all data across a business. While this is a lofty goal it is also a large investment over long periods of time. Lack of transparent prioritization and alignment to business needs (Pareto is a great tool for this) meant that stakeholders were unaware of why their work was not being completed yet the team was constantly busy.
- User Buy-in - Many organizations proceed down a path of consolidation dashboard technologies, while the cost savings and unification are positive outcomes the user base is often not bought-in and fearful of the impact it will have on their efficiency and productivity.
- Defined vs Executed Process - Many organizations that worked to unify their BI strategy and reporting failed to account for the inevitable manual workarounds that all organizations have for defined processes. BI dashboards and KPIs were built for processes as designed and failed to adjust for the manual interventions team members made daily to ensure business operations continued unimpeded, often working around limitations to systems or constraints from outside parties.
Each of these reasons emerges from the same underlying challenge, a gap in organizational literacy. The ability to apply past learnings to new technology and manage the complexities of driving adoption across an organization. We have a developing Data Literacy crisis due to the rapidly growing need to consume data effectively across an organization and execute on data-centric initiatives more quickly by enabling more teams to operate in parallel. This Data Literacy crisis is manifesting through four primary Data Literacy gaps:
- Data Consumption - Successful users are those who know where to locate data, and how to use the technology standardized to access the data and interpret the results in a way that drives positive change to process and business decisions.
- Trust - Data consumers will only leverage data and systems they trust. The foundation of Data Literacy programs is enabling users to understand where data comes from, the quality metrics it is measured by, and how to report non-compliant data for correction.
- Inflexible Technology Standards - Every organization sits somewhere on a spectrum of technology standards, with the end being absolute flexibility progressing through to orgs with a single uniform standard. Data Literacy programs ensure users are trained and required to use the technology available to them and know when and how to make decisions to bring in new tools to augment their work habits.
- Business User Input - Data Literacy programs are bi-directional, both rolling information out to users and listening to those users about future needs and enhancements. Users that feel heard become powerful champions for organizational priorities acting as change agents.
These gaps enable us to identify key learnings to apply for future data investments, ensuring Data Literacy is part of the plan:
- Most data-centric projects and investments fail due to adoption and consumption. Technology failure is rare. We must teach people to plan, test, and engage to ensure the organization is ready to adopt the capabilities that are built.
- Set expectations early with users and check in regularly to their perception. Ongoing engagement enables training plans to be tweaked, communication styles to be altered, and accommodations made for changing business conditions.
- Set a goal of enabling everyone in the organization to be an effective data consumer. Avoid the trap of limited access, limited licenses, and small training sessions. Make everyone a champion in the data-centric culture.
2024 is going to drive new expectations of our users. The consumerization of AI is driving awareness and ideation across all organizations on how to better consume and leverage data. This rapid adoption will drive several key focal points of adoption across an organization that we must both reinforce and manage the risk across the organization:
- Using Generative AI (GenAI) to create work breakdowns, accelerating planning for teams, and applying past best practices and lessons learned.
- The ability to identify where AI is being leveraged and intervene if data or results are incorrect. Teams apply a critical eye to results and are part of the process to improve.
- Accepting that technology will change rapidly, building a culture of shifting tech backed by enablement.
- Embedding technical resources with business teams to ensure alignment on priorities, rapid iteration, and the ability to develop depth on business processes and methods.
There are models across other aspects of technology that can assist us as we develop, evolve, and roll-out our Data Literacy programs. The cyber security domain has been highly effective at building training and programs that regularly reinforce specific skills, drive awareness of risks, and prompt user behavior around safe online activity. Cyber practitioners see this as an ongoing, often quarterly, time investment to ensure that all team members have a baseline of skills and the ability to go deeper into specific areas if desired. Cyber security training moves beyond the theoretical and into training that shows users how to configure specific services and protect themselves and their data while working remotely. These practices all enhance the uptake in training and increase effectiveness, providing effective models for Data Literacy programs to follow.
Effective Data Literacy, including strong foundations of data knowledge and communication, will be what make AI adoption successful. Data Literacy enables team members to effectively shift their working habits around new capabilities that AI brings and enables organizations to move past a history of failed data investments and initiatives through better understanding of requirements and user needs to build trust in data and consistent operating models.
Share this
You May Also Like
These Related Stories
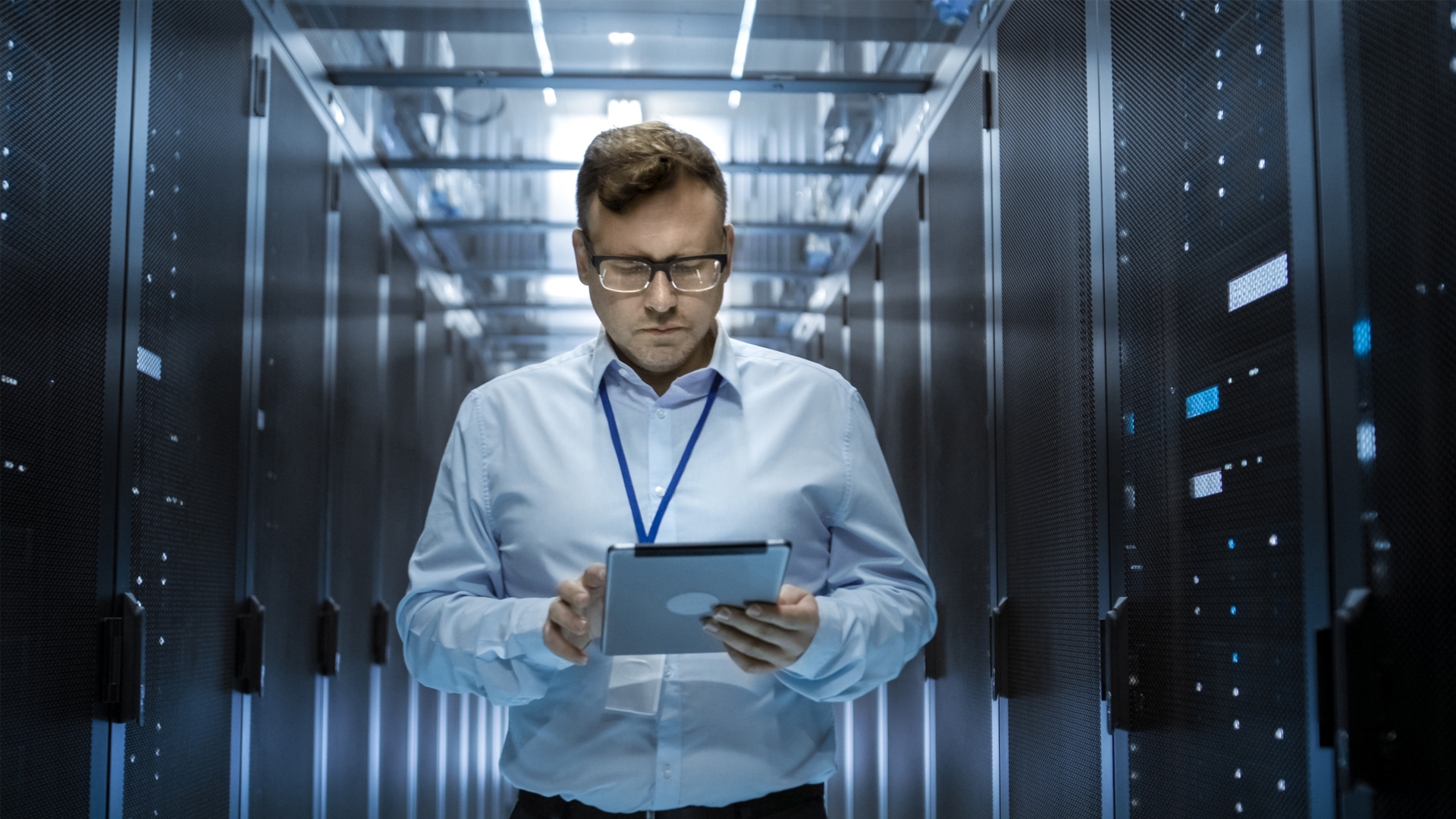
Data Literacy as an Accelerator
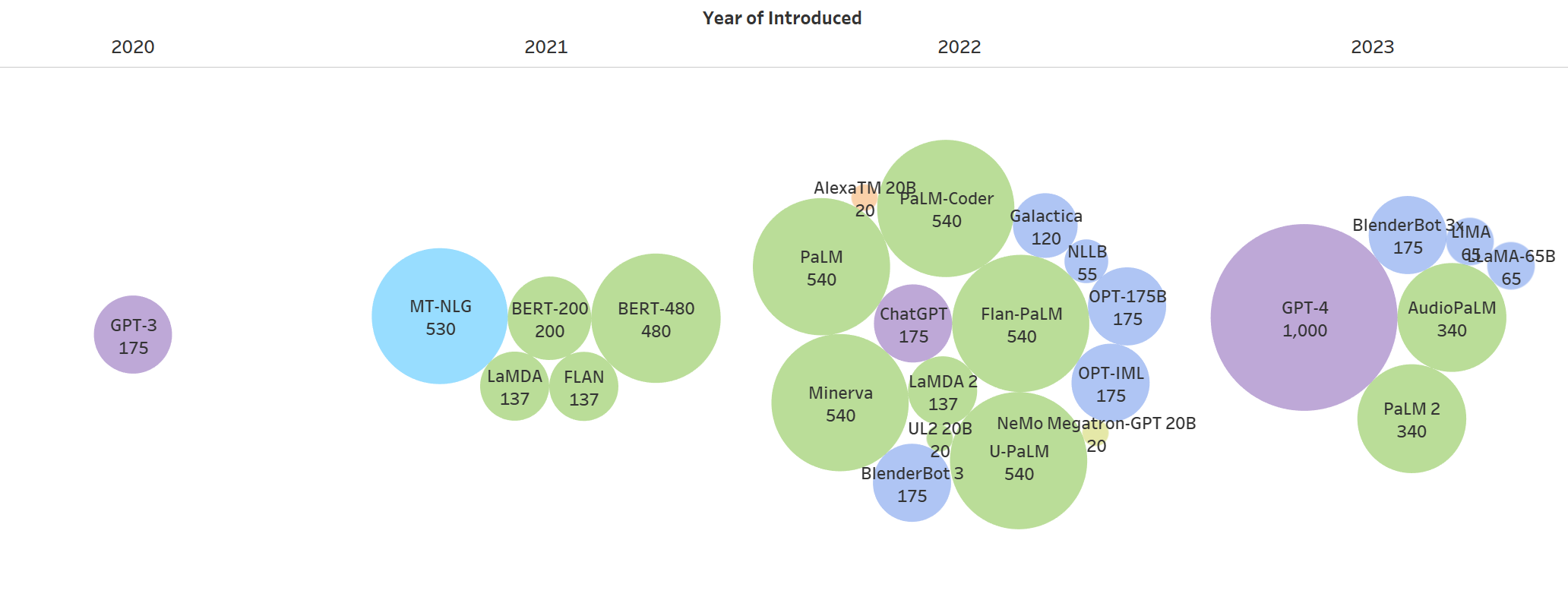
Harnessing the Power of GenAI: Advancements, Applications, and Strategies
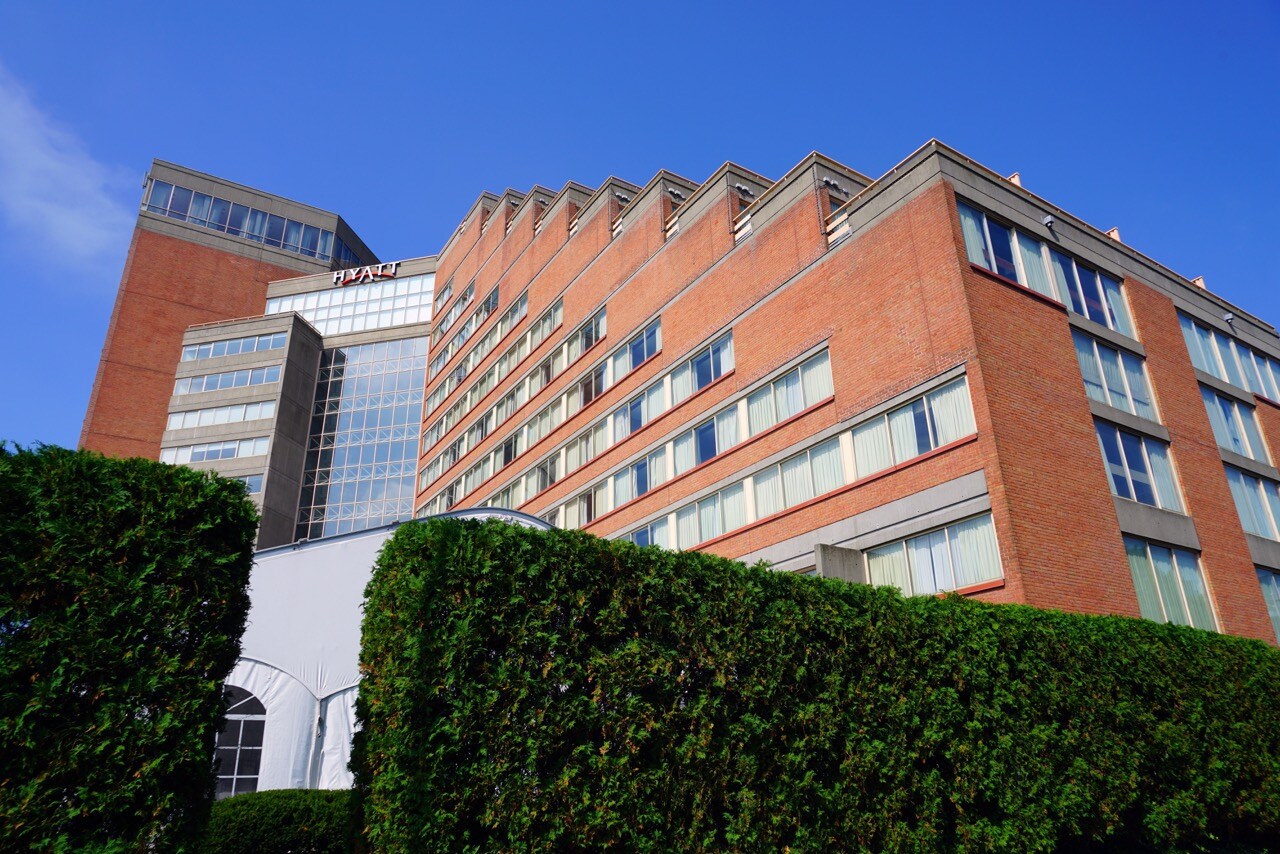
No Comments Yet
Let us know what you think