Predictive Analytics | Agribusiness
Commodities Firm Optimizes Bid Prices on High-Volume Transactions With Machine Learning
An agribusiness firm specializing in soybean exports and other commodities-related activities, the customer had more than a century of distinguished experience in the commodities industry. The company was looking to take advantage of all their historical data to optimize pricing models using predictive analytics and machine learning.

Overview
An agribusiness firm specializing in soybean exports and other commodities-related activities, the customer had more than a century of distinguished experience in the commodities industry. However, it needed to become more efficient and agile through technology to stay ahead of competitors and keep pace with today’s market realities.
That’s why the agribusiness firm’s leadership team approached Pythian for machine learning (ML) advice to help improve margins and sales team efficiencies. But they weren’t sure which use cases to pursue – while the company’s IT team boasted several very talented data scientists, Pythian was able to lend support by bringing our SMEs’ vast knowledge of ML solutions into the fold. It was also highly dependent on the corporate knowledge of a group of veteran sales people.
After attending a machine learning workshop organized by Pythian and conducting a risk/reward assessment of each idea, Pythian and the customer soon identified bid-acceptance prediction as the highest value use case.
What we did
Pythian and the customer used historical records of accepted bid prices and synthetically generated prices for rejected bids to create a proof of concept (PoC) model that predicts the probability of a given customer accepting or not accepting contracts, depending on the proposed price. The proof of concept model focused on a subset of the company’s products.
- Pythian first conducted a discovery and analysis phase to examine the quality of datasets used in the PoC and develop business rules for generating synthetic price data used in the predictive model.
- Pythian next imported the datasets into BigQuery and built a baseline model. This step included developing feature transformations (such as BigQuery preprocessing), creating synthetic data, and verifying synthetic data methods.
- A neural network model was then trained and improved through an iterative process that identified and designed architecture improvements and tuned the model for better performance.
Thanks to Pythian’s expertise in ML solutions and close relationship with Google, our SMEs, in collaboration with the agribusiness firm's internal team, developed a PoC that made sense for the company based on their understanding of the technology and the timelines and resources they needed to accommodate.
Key Outcomes
Pythian helped consolidate the company’s notable expertise into a tool that allows for lightning-fast decision-making, decision verification around price, and risk assessment, helping them scale their sales team and optimize workloads.
- The customer can now optimize their pricing on high-volume transactions based on data-driven probabilities while better recognizing the risk of not dropping the price in certain situations.
- The sales team can more easily scale by optimizing their workload and making more sales, while conducting transactions more confidently.
Pythian’s ML model architecture and design expertise, history of successfully tackling complex ML problems, and long partnership with Google ensured a successful project handoff. The ability to instantly see the last time a specific customer bought a product, at what price, and during which market conditions have already helped the customer improve their margins—and that’s just through a relatively limited PoC.
In the future, the agribusiness firm could enjoy similar cost optimizations across a broader range of products with further implementation of the PoC while relying on Pythian’s always available support services for AI and ML.
Reach your data transformation goals with an expert Google Cloud ML partner
Delve deeper into Google Cloud, ML, and AI
Learn more about the potential of Google Cloud ML services and the right Google Cloud ML partner. Check out our resources below:
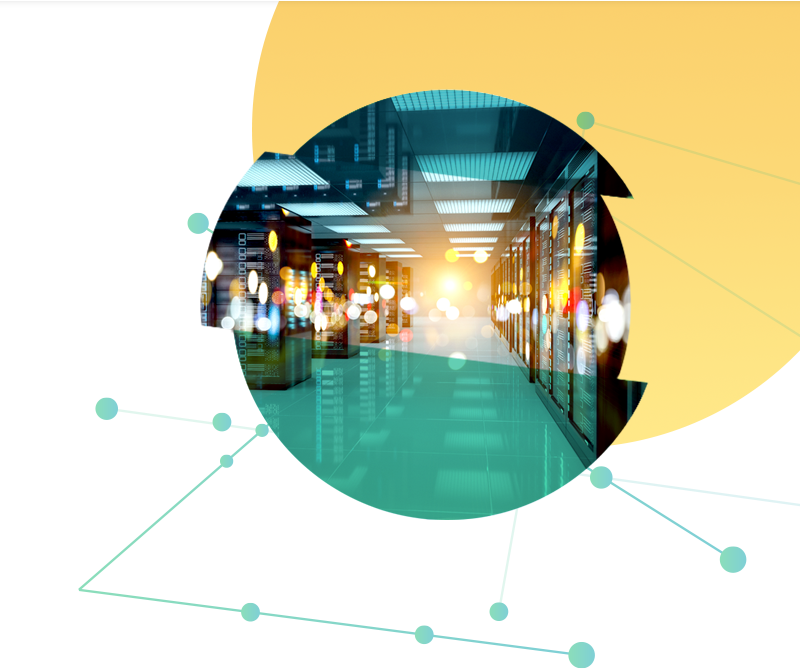
Next-Gen Supply Chain Solutions Provider Improves Search Accuracy Using GenAI
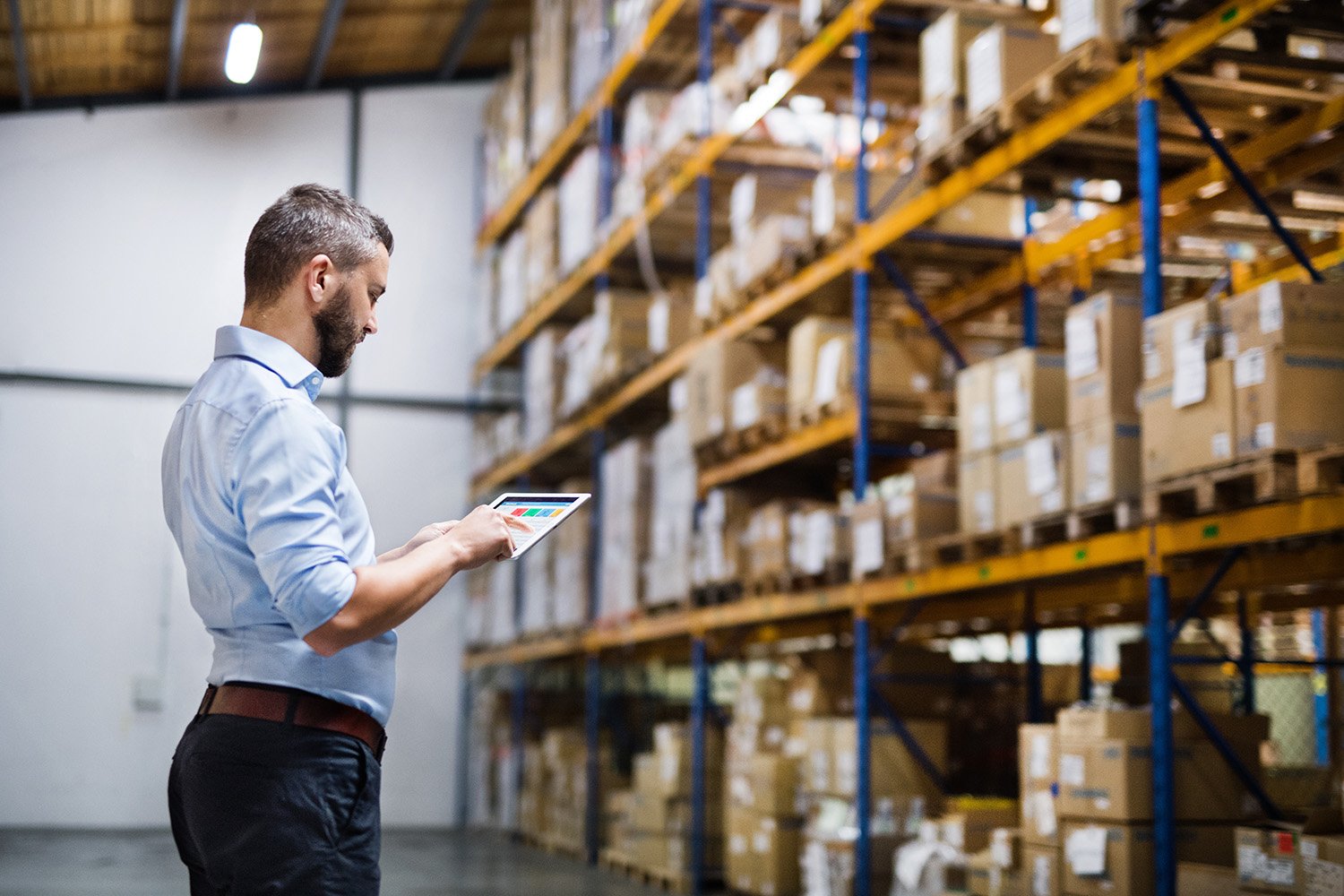
QAD achieves $1.7 million savings with Google Translate API and Machine Learning
