Big mining gets a boost from big data
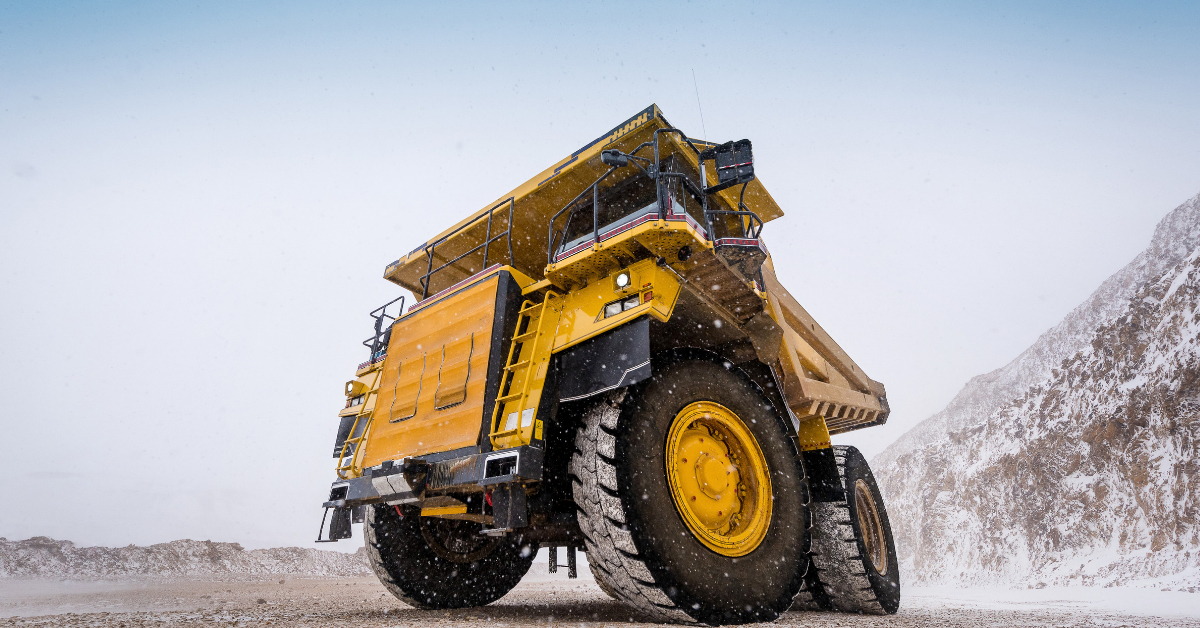
What do you do when the breakdown of a single vehicle could cost you a million dollars? Easy: you avoid breakdowns at all costs.
That’s exactly what Canada’s largest mining company is doing through a Google Cloud Platform artificial intelligence solution from Pythian.
The cost of downtime
When one of its 108 Komatsu 930E haul trucks has to be taken offline to fix a failure, Teck Resources incurs not only the cost of repairing the $1 million truck but also the much larger cost of downtime and lost productivity.
If only asset health managers at Teck’s three coal mining sites had an early warning system, maybe with estimated time to failure for each truck. Then they could pull the trucks from the line in time for scheduled preventive maintenance––much cheaper and less disruptive.
Enter Pythian.
Building the model
The Pythian project team’s first challenge was to create and train a model that would accurately predict high voltage electrical cabinet blow-ups––the most expensive and dramatic kind of failure. While IoT sensors on the Komatsu trucks’ thousands of components generate huge amounts of data, only a few worn-out parts might contribute to a breakdown.
How to narrow it down to the critical indicators? The team took two approaches.
First, it studied asset managers’ methods of observation and insight that have helped them ward off breakdowns for decades.
Second, it analyzed four years of structured data from sensors and alerts leading up to past breakdown and repair events. But since only 31 failures were available for analysis (some of them in the same machines; most trucks had no failures), the team had to extrapolate.
Predictions and payoffs
In the test round, the model predicted six electric cabinet failures on the haul trucks. Teck asset health managers actioned four of the predictions. The two that managers chose not to action actually went on to fail. And a seventh failure happened that was not predicted, either because thresholds were set too high or failure happened fast with no pattern.
The company was happy to get that rate of accuracy right off the bat. Since then, ROI has been higher than initially predicted. Teck’s CIO Kalev Ruberg: “From last April to this one, we saved $4 million from predicting and avoiding 10 truck failures.”
Accuracy drives adoption
Looking to create a successful model? Alex Gorbachev shared some best practices:
“Our team aimed for a model that generalized well without unnecessary complexity,” he said. “We kept trigger thresholds low to avoid false alarms and to help asset managers better trust the system. We also kept data clean through ‘yes’ and ‘no’ parameters, avoiding those ambiguous ‘maybes’.”
They integrated the AI solution with Teck’s processes through an API to help asset managers regularly query the system to find out which trucks need to be hauled in for maintenance.
“And, of course, we used Google Cloud Platform and Bigtable for the consistent, high-quality data needed for high-quality predictions,” Gorbachev concluded.
Hear Pythian’s Cloud CTO, Alex Gorbachev's presentation on two very different companies and how they created machine learning applications based on Google Cloud Platform. Save your seat now.
Share this
You May Also Like
These Related Stories
InnoDB error: Total number of locks exceeds the lock table size

No Comments Yet
Let us know what you think